DeGirum®
Cloud Platform
DeGirum® Cloud Platform revolutionizes the very approach to Edge AI development. From ML model design and application development to hardware selection and testing, it dramatically simplifies, shortens, and streamlines the development cycle.
DeGirum®
Cloud Platform
DeGirum® vs Traditional approach.
Deploy on Day One.

Traditional Approach
Investments in various HW
Multiple Application SW
Multiple Models for Each HW
Lost time, costs and opportunities
DeGirum® Approach
Only one application SW for all HW Options
Only one SW toolchain
Buying HW is the Last Step
Lightning-fast time to market. Saved time and costs
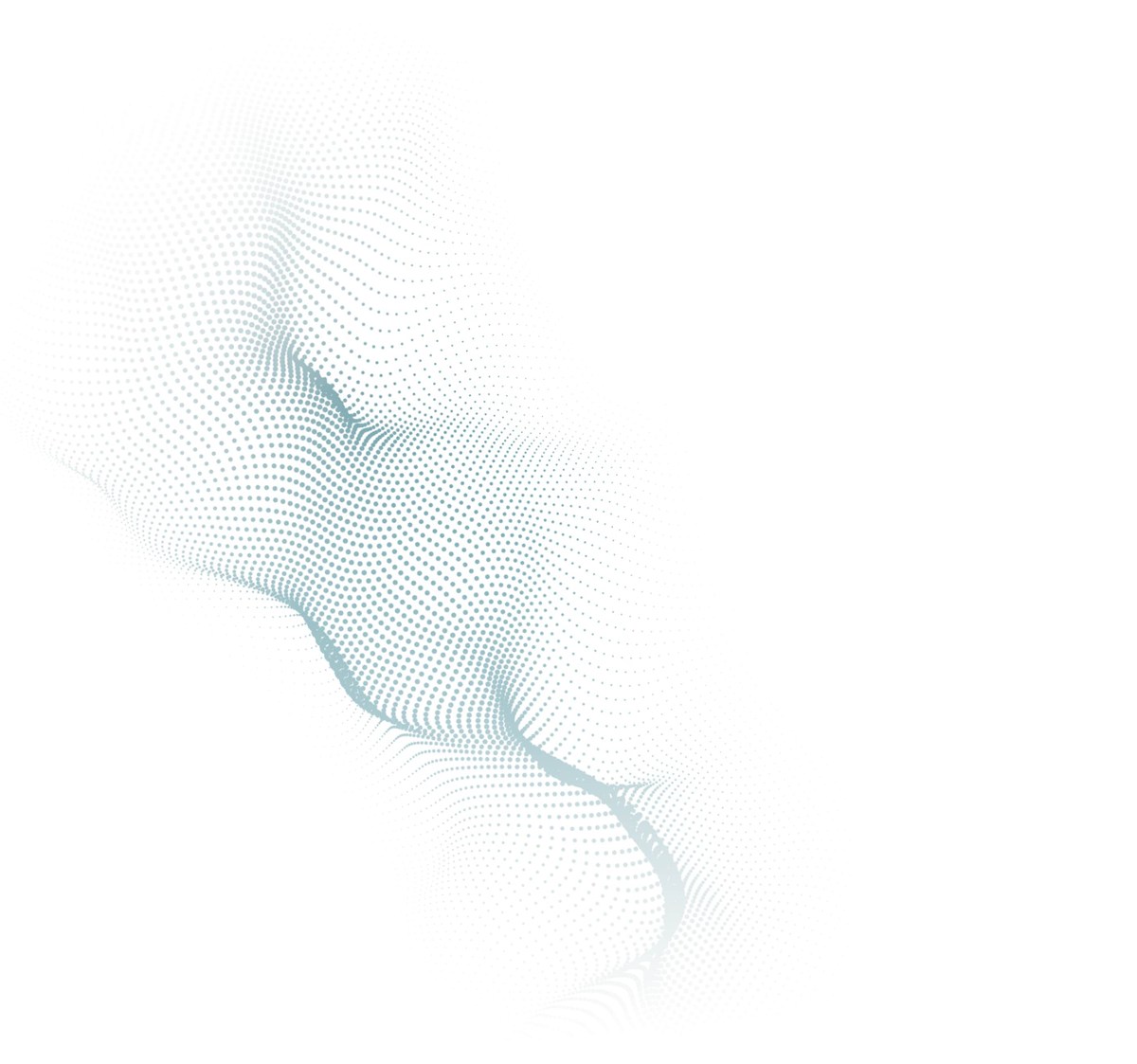
DeGirum®
Advantages
-
Powerful Hardware
DeGirum ORCA™ is a flexible, efficient, and affordable AI accelerator IC that delivers on metrics that matter.
-
Intuitive Software
DeGirum PySDK enables developing sophisticated AI applications with just a few lines of code.
-
Painless Model Integration
Integrate ML models using cloud compiler and evaluation tools.
-
Seamless Deployment
Manage deployed models and devices in the cloud
-
Evaluate before buy
Test your application on real hardware before investing in it